3 min read
The public launch of generative AI in late 2022 started a technology race that shows no sign of ending. The power of these tools, which depend on a type of AI known as a large language model, or LLM, is being recognized by businesses worldwide. LLMs can produce articles, analyze data, write computer code and more. But their computations require colossal amounts of energy.
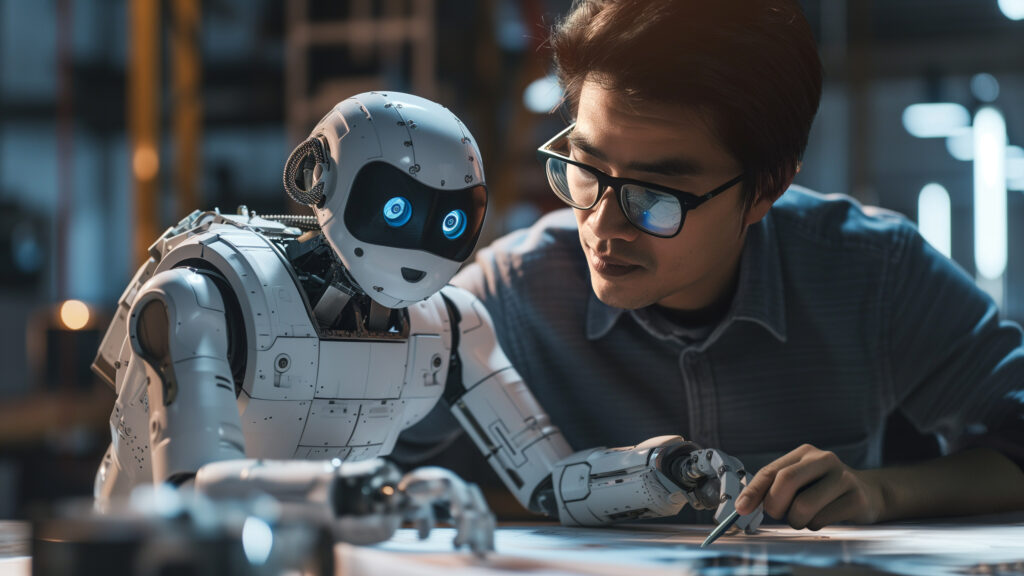
LLMs and Generative AI
LLMs are developed to analyze huge amounts of written data. This process, known as “training,” uses deep-learning algorithms to acquire a statistical understanding of the relationship between words. Knowing which words are likely to go together helps LLMs infer context to better understand natural language queries and produce high-quality written content themselves.
These are just one type of generative AI, a form of AI designed to create content. Other kinds use different models to produce images, video, audio and any other type of content. Between 2023 and 2030, the generative AI market is anticipated to experience exponential growth. By the end of 2023, it reached nearly $45 billion, almost twice its size in 2022. This trajectory, with an annual growth of nearly $20 billion, is projected to persist until the decade’s end, with the potential to increase global GDP by as much as 10%.
The technology can be used for a range of tasks, for example interpreting human sentiments to improve customer experience, providing immediate resolutions to supply chain bottlenecks, tailored medical diagnoses and recommendations, or interpreting complex graphs to accurately extract and contextualize information—and they can go beyond that.
Global technology company NTT is launching Tsuzumi, its own LLM designed to be hyper-efficient and therefore more sustainable. Work has already begun, implementing it in contact centers as well as in the finance and medical sectors, where it is contributing to operational efficiency and a better experience for employees and customers.
NTT’s focus is on the implementation of LLMs in various domains, including sustainability, corporate strategy and social well-being. The approach is to create a constellation of specialized AIs that work together to address both business needs and social issues at the same time, rather than relying on a single large-scale AI.
Flexible Capabilities
NTT’s Tsuzumi is an example of an ultra-light LLM. Even so, it has 600 million parameters—variables that define the characteristics and behavior of the AI model. Those parameters are adjusted as the model analyzes training data; more parameters typically mean more capabilities. This approach could provide solutions to complex problems from multiple perspectives without specialized expertise through small and specialized LLM collaboration.
The light version of Tsuzumi has seven billion parameters, still much smaller than most multi-purpose models, which can have as many as 175 billion. However, the more parameters, the higher the cost of running the hardware for each response. A smaller model is cost-effective, requires less electricity and with careful training to improve data quality, is very good at specialized tasks.
NTT’s vision of an AI constellation represents a paradigm shift to more distributed, specialized AI agents working collaboratively, rather than a single monolithic AI system. This approach could revolutionize how AI is deployed across multiple industries and sectors.
Reducing Power Consumption
A significant downside of monolithic LLMs is their power consumption. With many businesses trying to reduce energy use to reach net-zero emissions targets, generative AI could be challenging to implement without sacrificing operational efficiency. Tsuzumi demonstrates that an LLM flexibly customized for niche markets can achieve comparable results to a one-size-fits-all rival but at a fraction of the inference cost.
NTT achieves this by using lower-cost computer chips—graphics processing units, otherwise known as GPUs, or central processing units, CPUs. However, a more significant factor is the infrastructure behind these LLMs. NTT’s IOWN project will advance communications and computing infrastructure by integrating optical-based networks and devices that transmit information using light and traditional electronics.
This will allow data center networks to expand beyond the typical 60-kilometer limit. In time, optical connections will have extremely low latency between and within data centers, servers and chips—which will lead to a GPU cloud with low-performance degradation over long distances. This also enables the distribution of smaller data centers that are closer to renewable energy sources helping to contribute to a more sustainable AI infrastructure of the future.
GPUs for training LLMs can also be used more efficiently by reducing idle times through this synergistic infrastructure. Overall, this strategy is intelligent and pragmatic, addressing real-world business needs and societal challenges.